Insights
How Data Science and AI Are Changing Biometrics Careers
07 May, 202515 minutesBiometrics recruitment is shifting. The rise of AI and data science is altering the structur...
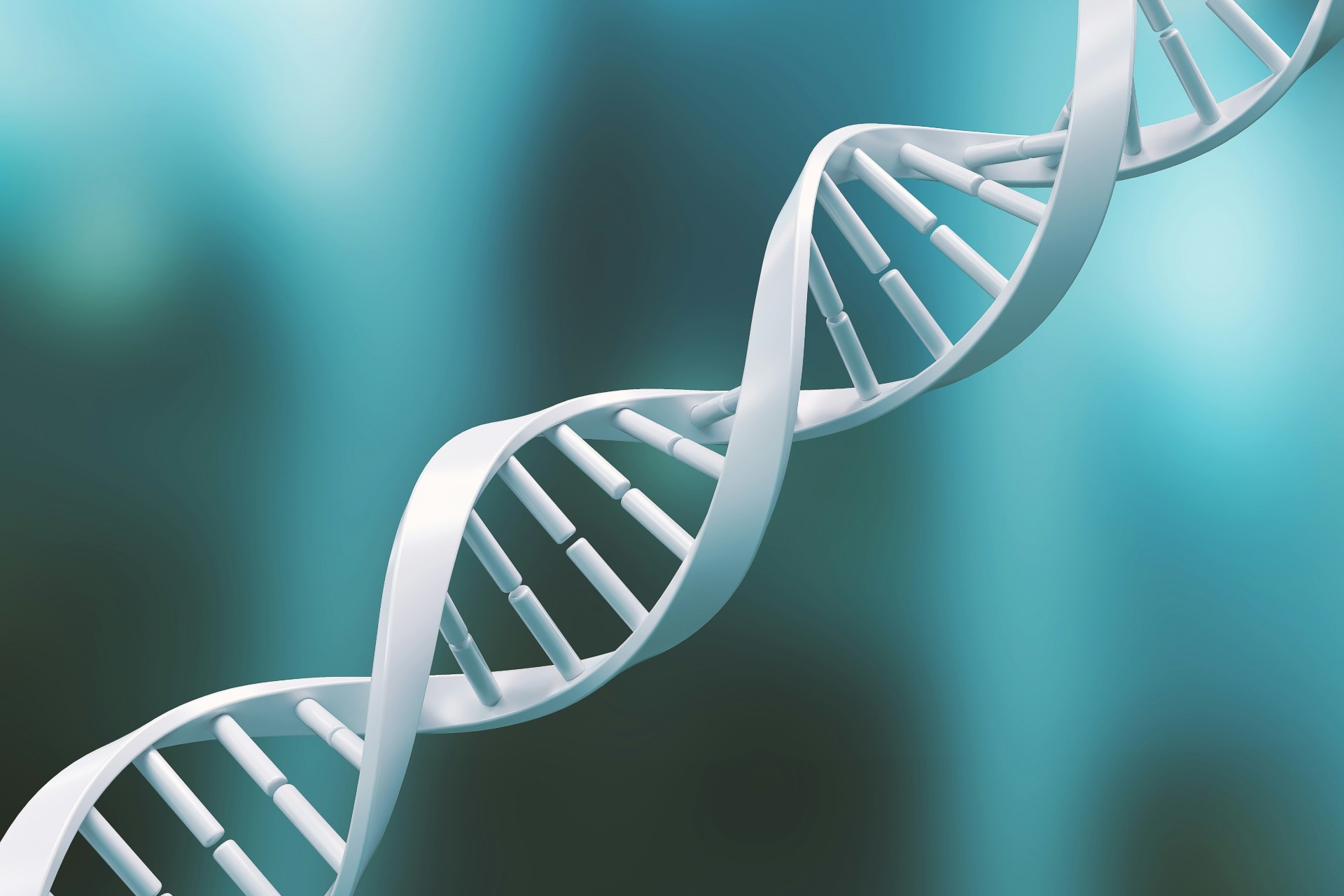
Biometrics recruitment is shifting. The rise of AI and data science is altering the structure of clinical and post-marketing teams, with growing demand for professionals who can operate across statistical programming, data integration, and machine-led analytics.
As organizations adopt more automated systems and predictive modeling tools, traditional biometrics roles are being redefined. Employers are placing more value on candidates with hybrid skills, exposure to newer platforms, and the ability to adapt to increasingly complex data environments.
In this blog, we explore how AI and data science are influencing biometrics careers, what this means for hiring, and how employers can respond.
The Shift in Biometrics Roles Driven by AI and Data Science
Over the past five years, data science has become a core part of biometrics delivery in clinical research. The combination of larger real-world datasets, decentralized trial models, and more complex study designs has pushed biometrics teams to adapt. Programming, modeling, and analysis are no longer treated as downstream tasks. Instead, they are now integrated across planning, oversight, and regulatory delivery.
At the same time, artificial intelligence is being used to reduce manual burden, support accuracy, and standardise decision-making. These tools are not designed to replace biostatistics or data management teams. They support consistency, accelerate review processes, and help biometrics teams meet higher expectations for traceability and data confidence.
Where data science and AI are currently used in biometrics:
- Predictive models to support patient enrollment planning and reduce dropout risk.
- Simulation tools for protocol design and endpoint strategy.
- Natural language processing applied to free-text clinical notes, SAE reports, and external data.
- Query prioritization systems in clinical data management to reduce review timelines.
- Real-time statistical checks on large datasets ahead of database lock.
- Integration of real-world evidence with study data to inform signal detection and long-term outcomes.
- Programming across Python, R, and SAS to meet different data and automation requirements.
According to HealthTech Magazine, more than 30% of global healthcare data now comes from real-world sources such as EHRs, mobile apps, and patient monitoring platforms. For biometrics teams, this shift has introduced new demands around data standardization, source traceability, and audit readiness. Inconsistent outputs, undocumented edits, or unclear data lineage can cause delays during submission or raise concerns during inspection.
While tools have improved, the volume and complexity of data continue to increase. This has raised the level of technical oversight and documentation required across every part of the biometrics function.
AI in the Biometrics Workflow: Efficiency and Accuracy Gains
AI is not redefining biometrics, but it is influencing how key tasks are completed within high-pressure delivery environments. Across clinical and post-approval studies, its application is becoming more focused, supporting teams with structured validation, targeted review, and data consistency at scale.
These systems are not applied across the board. Most are configured to support specific parts of the biometrics workflow, such as field-level discrepancy checks, metadata reconciliation, or real-world data formatting. Their effectiveness depends on how well they are aligned with protocol expectations, submission timelines, and the surrounding infrastructure.
In the sections that follow, we examine how AI is being applied across different stages of biometrics delivery and what this means for the skills, oversight, and technical depth now expected within biometrics recruitment and delivery teams.
Cleaning biometric data at volume
In decentralized or late-phase studies, inconsistency in biometric inputs creates high-volume cleaning backlogs. Site variation, fragmented device data, and protocol logic discrepancies often lead to thousands of low-priority queries. AI applications within CDMS platforms are being used to focus cleaning on the most submission-relevant issues.
Current uses include:
- Reviewing longitudinal vitals to flag deviation trends at subject or site level
- Identifying broken data sequences in device or ePRO submissions
- Classifying queries based on their likely impact on regulatory endpoints
These functions reduce manual lift without replacing CDM judgement. For clinical data science jobs, being able to focus only on the most significant inconsistencies is key to protecting lock timelines.
Programming validation before QC
Statistical programmers are using AI-supported checks to confirm that SDTM and ADaM datasets meet structural and metadata requirements before final QC. These systems flag broken mappings, incomplete derivations, or discrepancies introduced during mid-study updates.
Applied use cases include:
- Verifying dataset format against CDISC structure and metadata
- Identifying unmapped or redundant variables
- Flagging breaks in variable lineage across raw and derived datasets
These checks are particularly valuable in complex studies or when multiple analysis populations are being run in parallel. They reduce rework, not oversight.
Database lock and late-phase delivery
As trials approach lock, AI is used to surface unresolved issues that would otherwise delay timelines. Rather than running new checks, these systems highlight risks based on audit trails, query trends, and field completeness.
Examples include:
- Flagging unresolved data states across interim and final datasets
- Identifying inconsistencies in submission-critical variables
- Alerting teams to version drift across multi-lock environments
This helps biometrics teams reallocate programming and CDM support where it matters most, before timelines are affected.
Post-marketing and real-world evidence handling
In post-approval studies, AI is used to structure real-world data so it can be analysed or submitted without compromising traceability. Data from registries, EHRs, or patient apps often lacks standardization and must be reconciled before analysis begins.
Common uses include:
- Formatting RWD to align with SDTM-like structures
- Running duplication checks across multi-source records
- Producing traceability logs for transformed datasets
In biostatistics jobs tied to long-term follow-up or HTA support, verifying AI-transformed outputs is now a common part of biometrics delivery.
Skills Biometrics Professionals Need in 2025 and Beyond
AI systems now support core data tasks across clinical studies. They flag issues, apply standardization, and generate derived outputs. Biometrics teams are responsible for checking those outputs, understanding how they were built, and ensuring they meet protocol and regulatory standards.
The focus of biometrics recruitment has shifted to interpretation, validation, and system-level oversight. Candidates are expected to bring:
- Programming fluency across SAS, Python, R, and SQL
- Experience with cloud tools like AWS or GCP
- Familiarity with ML libraries such as TensorFlow and scikit-learn
- Confidence reviewing AI-derived outputs in a regulatory context
- Skills to manage structured and unstructured data across multiple sources
The next section outlines five technical skill areas shaping hiring across statistical programming jobs, biostatistics jobs, and clinical data science roles.
1. Diagnostic scripting and derivation troubleshooting
As clinical trial automation accelerates, statistical programmers and biostatisticians are expected to interrogate flagged issues rather than just generate outputs. This means writing targeted scripts that trace variable logic across SDTM and ADaM, comparing derivations across database cuts, and identifying where pre-processed outputs diverge from protocol expectations.
What to look for:
- SAS and Python fluency for cross-environment testing
- Experience debugging AI-altered variables or mappings
- Strong documentation habits to support traceability in regulatory submissions
This skillset is now standard across high-spec statistical programming jobs.
2. AI-informed statistical judgement
Variables generated through machine learning or NLP tools are increasingly being used in trial analysis. Biostatisticians must know how those variables were derived, evaluate whether they align with the SAP, and assess how they affect trial populations or endpoints.
The ability to challenge model assumptions or identify misclassification is critical to delivery.
What to look for:
- Biostatistics jobs with prior exposure to model-derived data
- Experience reviewing feature sets, training documentation, and model consistency
- Confidence in escalating outputs that are not statistically or clinically reliable
This is becoming a core requirement in biometrics careers that involve AI in clinical trials.
3. Metadata control and transformation oversight
Automated formatting tools save time, but errors in metadata can still compromise submission timelines. Teams must be able to maintain consistent naming conventions, manage version histories, and confirm that variable lineage is clear and auditable from source to submission.
What to look for:
- Clinical data managers experienced in mapping EDC outputs and managing terminology
- Programmers who manage structured metadata and transformation logs
- Statisticians with an understanding of dataset history and structural integrity
These capabilities are now key to biometrics jobs that involve multi-phase studies or complex submissions.
4. Risk-led review and query strategy
Automated checks can generate high volumes of queries. The value now lies in being able to triage based on risk, prioritizing fields that impact protocol endpoints, safety data, or eligibility criteria. Teams must assess both the severity and context of flagged data, not just its frequency.
What to look for:
- CDMs who assess cleaning cycles by clinical importance, not order of appearance
- Programmers who interpret trend-level query clusters rather than individual rows
- Team structures that support escalation based on impact
This risk-focused mindset is becoming more important as AI is used to manage large, decentralized datasets.
5. Real-world and unstructured data validation
Real-world evidence and post-marketing studies often rely on data processed through AI platforms. These datasets arrive formatted, but are rarely verified. Biometrics teams must be able to check that transformations reflect real clinical meaning, align with protocol definitions, and meet regulatory expectations for traceability.
What to look for:
- Experience reviewing NLP or sensor-derived data mapped into clinical structures
- Familiarity with merging CRF data and external registries in RWE studies
- Statisticians or programmers who can validate model-classified variables before use in reporting
As more clinical data science jobs include responsibility for real-world data integration, this is becoming a recruitment priority.
How Employers Should Respond to Changing Biometrics Skill Needs
AI is now integrated into core biometrics processes, but its use brings regulatory weight. In the US, the FDA’s draft guidance on AI in clinical trials and 21 CFR Part 11 emphasize validation, auditability, and oversight. In the EU, the AI Act and GDPR set clear limits on how biometric and clinical data can be processed. These standards affect hiring decisions directly.
Employers must prioritize people who understand regulated systems, and restructure internal processes to support traceability and inspection readiness from the start.
Be precise about what roles need to support automation:
Not every hire needs to be an AI expert. But in biostatistics, clinical data management, and statistical programming, every role does now needs to function alongside automated systems. This means reframing job descriptions to reflect real delivery responsibilities:
- Statistical Programmers: Should be able to review and validate outputs from AI-supported discrepancy detection systems or algorithm-based edit checks.
- Biostatisticians: Increasingly expected to collaborate with data scientists to assess ML-driven models and align them with protocol endpoints.
- Clinical Data Managers: Must understand how AI systems flag anomalies or trends, and know how to document overrides, exclusions, and data changes in a regulated format.
These details matter. Many CVs mention tools like Python or ML libraries. What matters is proven responsibility in controlled, validated environments.
Upskill around traceability, not just tooling:
AI adoption has introduced new documentation risks. It is no longer enough to run a clean database. Teams must be able to show how model-based decisions were made, which outputs were adjusted, and why.
Support this by offering:
- Training on audit trail review, AI output validation, and documentation of overrides
- Joint sessions between data managers, programmers, and QA to refine SOPs for AI-integrated systems
- Practical workshops on tools used for predictive enrollment or endpoint optimisation, focusing on traceable output and compliance
Work with recruiters who understand regulated automation:
Generalist recruiters may not understand the regulatory weight behind AI-influenced biometrics jobs. Hiring managers need support from partners who can assess not just skills, but systems accountability.
Look for agencies that:
- Know what validated AI experience looks like across biometrics functions
- Can identify candidates who’ve supported SDTM or ADaM outputs generated through semi-automated systems
- Offer shortlist support that includes regulatory context, not just tool familiarity
Make it clear why the best candidates should choose you:
The strongest biometrics professionals are being approached daily. Those with SAS and R, cross-functional experience, or ML project exposure are commanding premium offers. But what they want is more than salary.
Make sure your EVP communicates:
- Opportunities to shape SOPs and system design for AI-supported workflows
- Access to diverse trial phases, including real-world evidence and post-marketing analytics
- Investment in long-term career development tied to compliance and innovation
The market is competitive, but clarity wins. You become the employer of choice when you show candidates that their role is critical to both innovation and regulatory delivery.
Final Thoughts on Biometrics, Recruitment, and Data Transformation
AI and data science are now embedded in biometrics delivery. From patient recruitment models to real-world data structuring, these tools are changing how teams operate and what employers expect. However, systems alone cannot meet regulatory standards or ensure inspection readiness.
Hiring decisions need to reflect this shift. The most effective biometrics teams combine strong programming and analytical skills with a clear understanding of how automated outputs must be validated, interpreted, and documented.
With new demands across clinical data science, statistical programming, and biostatistics jobs, success depends on early planning, role alignment, and specialist hiring support.
Build a Biometrics Team That Delivers
Since 2017, we have supported biotech and CRO partners with recruitment across biostatistics, clinical data management, and statistical programming. From contract planning to regulatory submission, our consultants offer the insight and precision to hire confidently in complex, regulated environments.
Get in touch to find out how we can support your next hire or strengthen your biometrics delivery.